

- Paraview label format integer how to#
- Paraview label format integer registration#
- Paraview label format integer license#
Paraview label format integer license#
mhd) format with a Creative Commons Attribution 3.0 United States license which pretty much translates to “you can do whatever you want with this”. The datasets are distributed in a zipped MetaImage (.
Paraview label format integer registration#
The RIRE Project provides patient datasets acquired with different imaging modalities, e.g., MR, CT, PET, which are meant to be used in evaluation of different image registration and segmentation techniques. Today’s dataset is taken from the Retrospective Image Registration Evaluation (RIRE) Project, which was “designed to compare retrospective CT-MR and PET-MR registration techniques used by a number of groups”. While the typical multi-modal segmentation involves combining CT and MR images (thus getting a great depiction of bones and soft tissue), I decided to keep this simple and instead show a case of gray matter segmentation based on the combination of T1 and T2 images of the same anatomy. Multi-modal segmentation essentially leverages the fact that we have multiple images of the same anatomy and allows for more precise and often faster segmentation of an anatomy. Such a case is the gray matter which appears darker than white matter in T1-weighted images but considerably brighter than white matter in T2-weighted images. In addition, differently weighted images and different contrast agents depict the same anatomies with different intensities. On the other hand, magnetic resonance (MR) images provide excellent contrast in soft-tissue, e.g., muscle, brain matter, etc, anatomies barely visible with CT. However, different imaging modalities give us very different images of the same anatomy.įor example, a computed tomography (CT) dataset would provide a very clear depiction of bone structures in the human body.
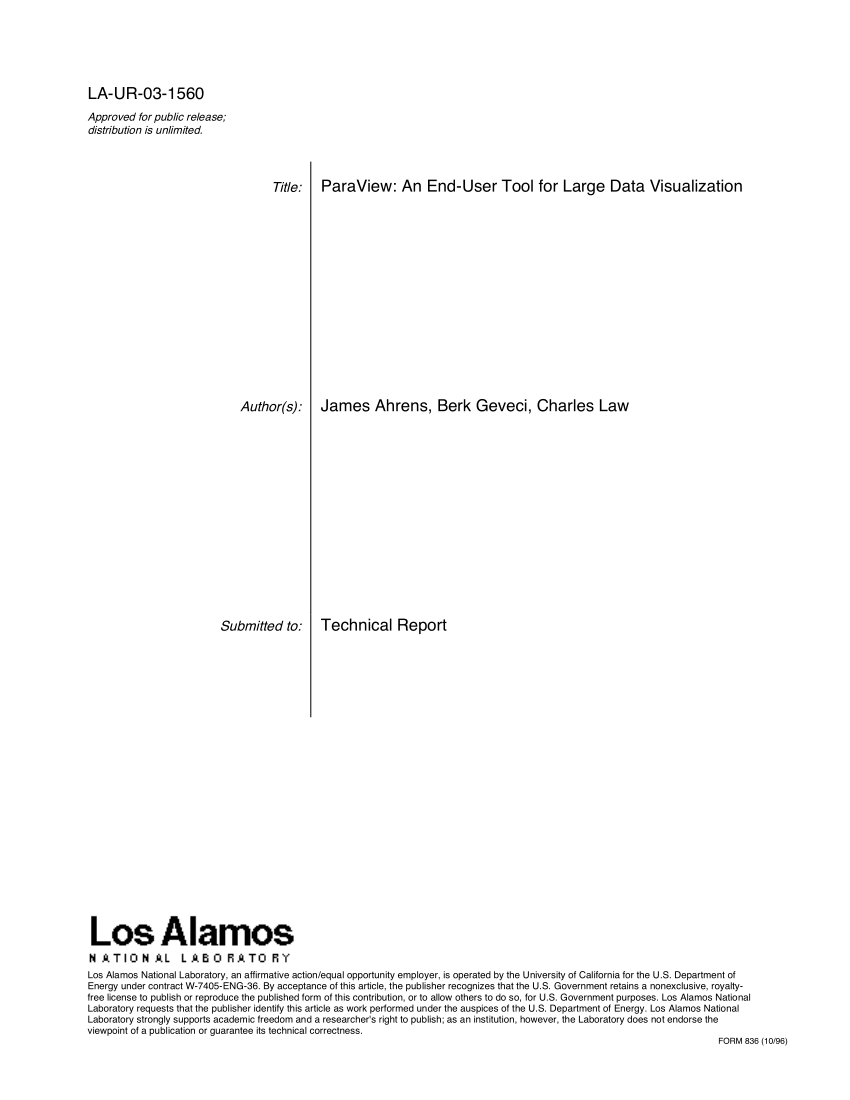
Typically, when performing segmentations of medical image data we use a single dataset acquired through a single imaging modality, e.g., MRI, CT, PET, etc. If you haven’t read that post I recommend that you do so before proceeding with this one as I will not be re-visiting these topics. The primary strengths of SimpleITK, links and material, as well as its installation – for vanilla and alternative Python distributions – was extensively discussed in the last post entitled ‘Image Segmentation with Python and SimpleITK’. In the previous post, I introduced SimpleITK, a simplified layer/wrapper build on top of ITK, allowing for advanced image processing including but not limited to image segmentation, registration, and interpolation. The tutorial will include input and output of MHD images, visualization tricks, as well as uni-modal and multi-modal segmentation of the datasets.
Paraview label format integer how to#
In this post I will show how to use SimpleITK to perform multi-modal segmentation on a T1 and T2 MRI dataset for better accuracy and performance.
